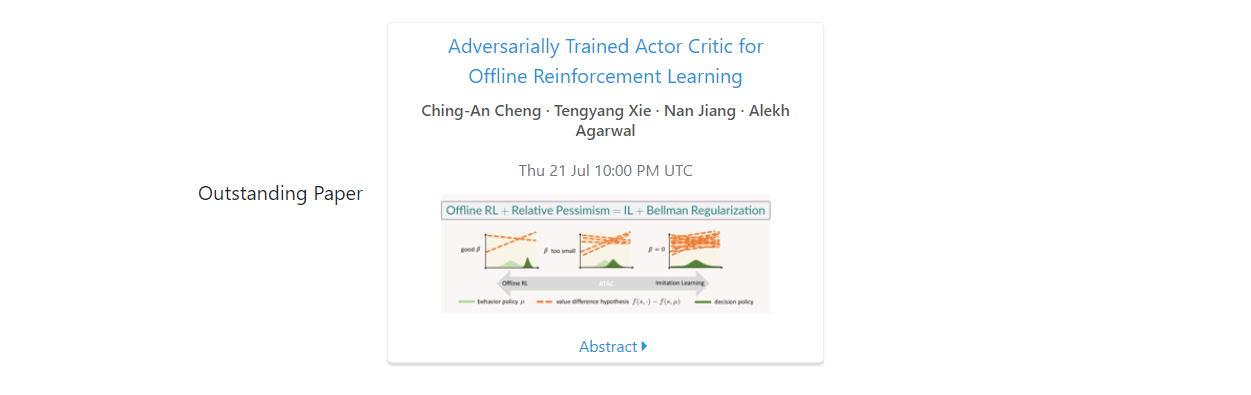
Adversarially Trained Actor Critic for Offline Reinforcement Learning
Pdf: https://arxiv.org/pdf/2202.02446.pdf
Github: https://github.com/microsoft/ATAC
We propose Adversarially Trained Actor Critic (ATAC), a new model-free algorithm for offline reinforcement learning (RL) under insufficient data coverage, based on the concept of relative pessimism. ATAC is designed as a two-player Stackelberg game: A policy actor competes against an adversarially trained value critic, who finds dataconsistent scenarios where the actor is inferior to the data-collection behavior policy. We prove that, when the actor attains no regret in the twoplayer game, running ATAC produces a policy that provably 1) outperforms the behavior policy over a wide range of hyperparameters that control the degree of pessimism, and 2) competes with the best policy covered by data with appropriately chosen hyperparameters. Compared with existing works, notably our framework offers both theoretical guarantees for general function approximation and a deep RL implementation scalable to complex environments and large datasets. In the D4RL benchmark, ATAC consistently outperforms state-of-the-art offline RL algorithms on a range of continuous control tasks.
我们基于相对悲观的概念提出了对抗训练的演员评论家(ATAC),这是一种在数据覆盖不足的情况下用于离线强化学习(RL)的新的无模型算法。 ATAC 被设计为两人的 Stackelberg 游戏:策略参与者与经过对抗训练的价值评论家竞争,后者发现参与者不如数据收集行为策略的数据一致场景。我们证明,当参与者在双人游戏中没有后悔时,运行 ATAC 产生的策略可证明:1)在控制悲观程度的广泛超参数上优于行为策略,以及 2)与涵盖的最佳策略竞争by data with appropriately chosen hyperparameters.与现有工作相比,值得注意的是,我们的框架既为一般函数逼近提供了理论保证,又为可扩展到复杂环境和大型数据集的深度 RL 实现提供了保障。在 D4RL 基准测试中,ATAC 在一系列连续控制任务上始终优于最先进的离线 RL 算法。
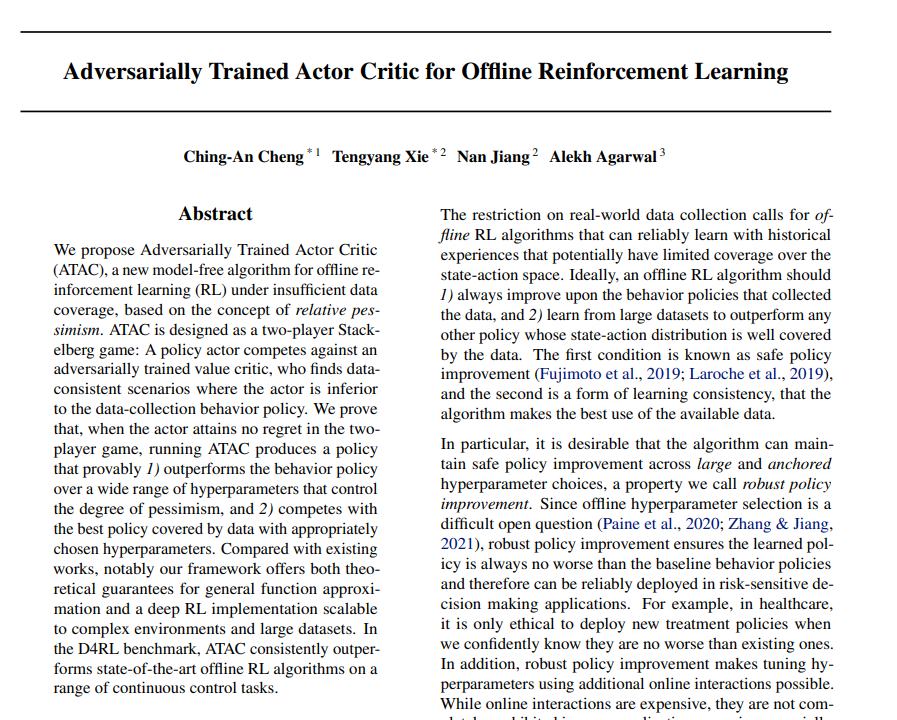
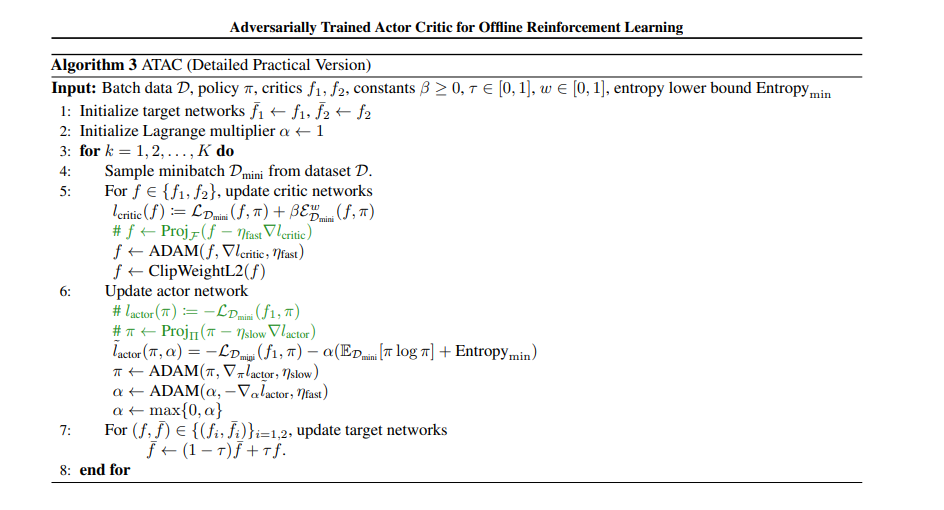