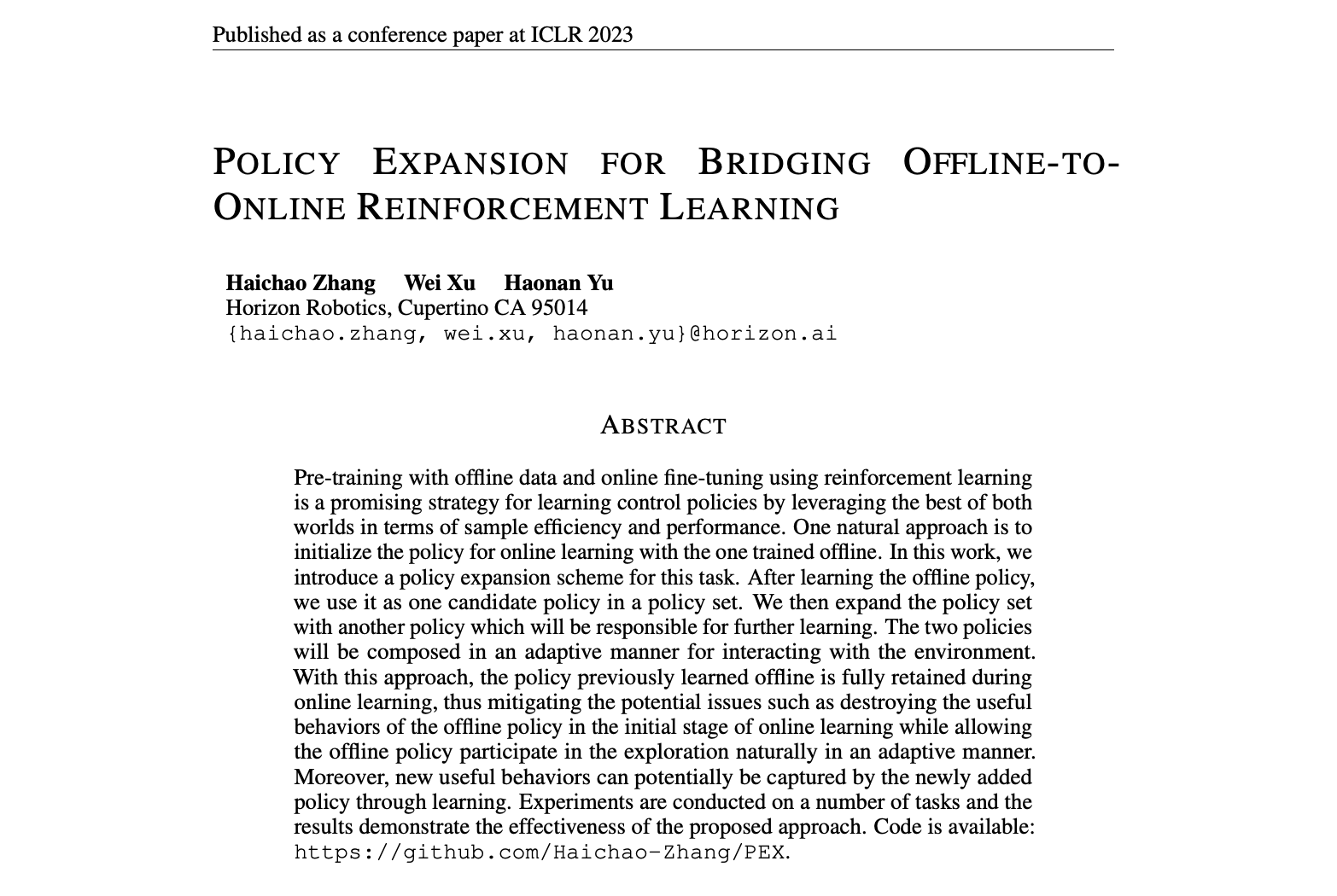
Abstract: Pre-training with offline data and online fine-tuning using reinforcement learning is a promising strategy for learning control policies by leveraging the best of both worlds in terms of sample efficiency and performance. One natural approach is to initialize the policy for online learning with the one trained offline. In this work, we introduce a policy expansion scheme for this task. After learning the offline policy, we use it as one candidate policy in a policy set, and further learn another policy that will be responsible for further learning as an expansion to the policy set. The two policies will be composed in an adaptive manner for interacting with the environment. With this approach, the policy previously learned offline is fully retained during online learning, thus mitigating the potential issues such as destroying the useful behaviors of the offline policy in the initial stage of online learning while allowing the offline policy participate in the exploration naturally in an adaptive manner. Moreover, new useful behaviors can potentially be captured by the newly added policy through learning. Experiments are conducted on a number of tasks and the results demonstrate the effectiveness of the proposed approach.
:
Background:
a. Subject and characteristics:
- 本文论述了在强化学习(RL)中,离线数据如何影响在线学习和策略更新,并提出了一种策略扩展方案以有效地学习策略。
b. Historical development
c. Past methods
d. Past research shortcomings
e. Current issues to address
Methods:
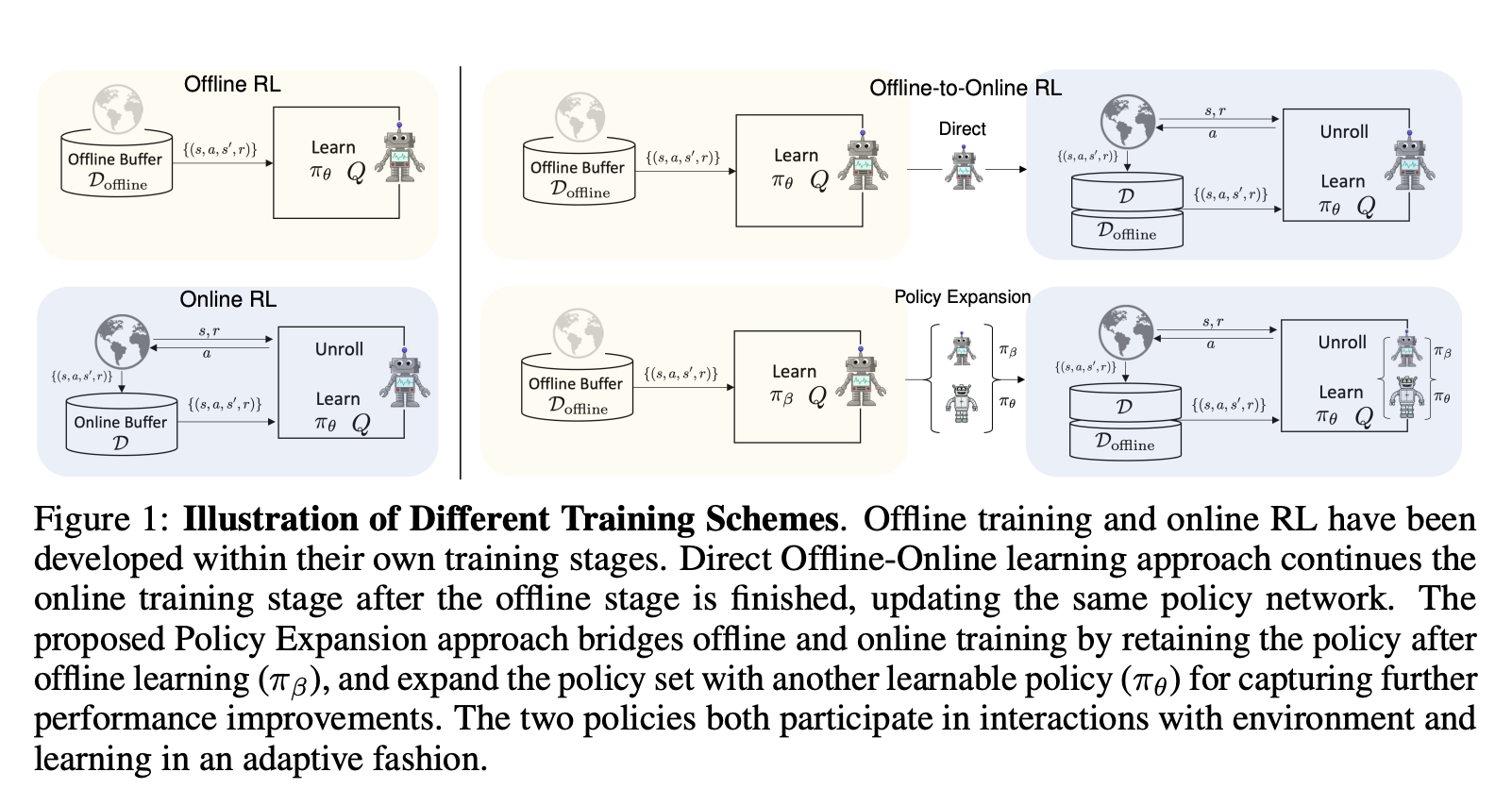
Conclusion:
a. Work significance
- 本研究提出了一种能够更好地学习策略并有效集成到现有算法中的方案,提高了算法的灵活性和性能,使RL能够更加广泛地应用于实际场景。
b. Innovation, performance, and workload
c. Research conclusions (list points)
本文提出一种离线和在线RL的互补性方法,即策略扩展方案(PEX)。
策略扩展方案利用已离线训练策略和另一个策略进行进一步学习,提高了算法的灵活性和性能。
策略扩展方案解决了在线学习中已有策略被破坏的潜在问题,并允许其自然地参与探索。同时,新添加的策略也可以通过学习捕获新的有用特征。
策略扩展方案在各种任务和环境设置下取得了良好的性能表现,并且与现有的基线相比具有更好的性能和样本效率。
@inproceedings{
zhang2023policy,
title={Policy Expansion for Bridging Offline-to-Online Reinforcement Learning},
author={Haichao Zhang and Wei Xu and Haonan Yu},
booktitle={The Eleventh International Conference on Learning Representations },
year={2023},
url={https://openreview.net/forum?id=-Y34L45JR6z}
}