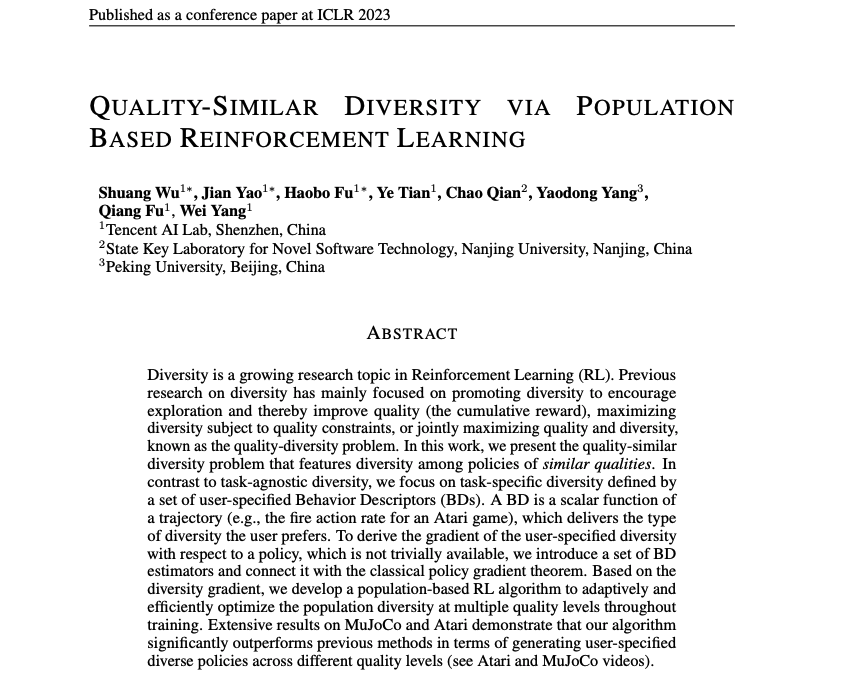
Abstract: Diversity is a growing research topic in Reinforcement Learning (RL). Previous research on diversity has mainly focused on promoting diversity to encourage exploration and thereby improve quality (the cumulative reward), maximizing diversity subject to quality constraints, or jointly maximizing quality and diversity, known as the quality-diversity problem. In this work, we present the quality-similar diversity problem that features diversity among policies of similar qualities. In contrast to task-agnostic diversity, we focus on task-specific diversity defined by a set of user-specified Behavior Descriptors (BDs). A BD is a scalar function of a trajectory (e.g., the fire action rate for an Atari game), which delivers the type of diversity the user prefers. To derive the gradient of the user-specified diversity with respect to a policy, which is not trivially available, we introduce a set of BD estimators and connect it with the classical policy gradient theorem. Based on the diversity gradient, we develop a population-based RL algorithm to adaptively and efficiently optimize the population diversity at multiple quality levels throughout training. Extensive results on MuJoCo and Atari demonstrate that our algorithm significantly outperforms previous methods in terms of generating user-specified diverse policies across different quality levels.
:
Summary:
(1): 本文针对强化学习中的任务特异性差异性问题提出了一种新的方法,称为Quality-Similar Diversity (QSD)。通过引入用户指定的行为描述符(Behavior Descriptors,BDs)来定义任务特定的差异性,并基于这些差异性开发一种基于群体的强化学习算法来在训练过程中优化不同质量水平下的群体差异性。
(2): 过去的差异性研究主要旨在提高累积奖励或在质量约束条件下最大化差异性,但QSD问题的特点是质量相似的政策之间的差异性。为此,作者引入了一组用户指定的BDs来定义任务特定的差异性。作者提出了一组BD估计器,用于预测当前策略的BD值,并使用策略梯度定理导出用户指定的BDs相对于策略的梯度。基于差异性梯度,他们开发了一种基于群体的RL算法,可以在训练过程中优化多个质量水平的群体差异性。 QSD-PBT算法的性能优于以前的方法,可以在MuJoCo和Atari环境中生成用户指定的不同质量水平下差异性的策略,并且有效地优化了一些实际应用中优先考虑非最优质量策略的差异性。
(3): 本文提出的方法包括:定义QSD问题,引入了一种新的QD度量标准,导出了指定BDs相对于策略的梯度,开发了一种基于群体的RL算法,并展示它在不同质量水平下生成用户指定的不同策略差异性方面的有效性。
(4): 本文的方法可以生成用户指定的差异策略,在多种环境中实现了任务特定的多样性。度量标准表明 QSD-PBT可以在许多任务中生成视觉上不同的策略,与以前的方法相比具有更好的性能。
Background:
a. Subject and characteristics:
- 本文的主题为强化学习中的任务特定差异性。强化学习是一种智能系统能够从环境中学习的框架。差异性是指强化学习算法在学习过程中产生的不同的策略。
b. Historical development:
c. Past methods:
d. Past research shortcomings:
e. Current issues to address:
Methods:
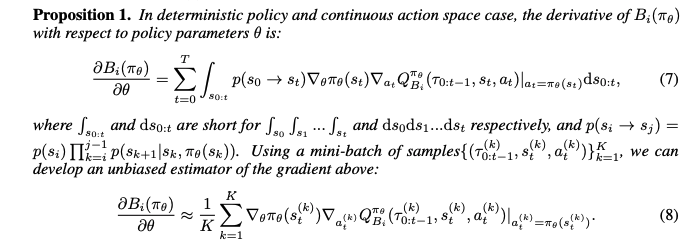
Conclusion:
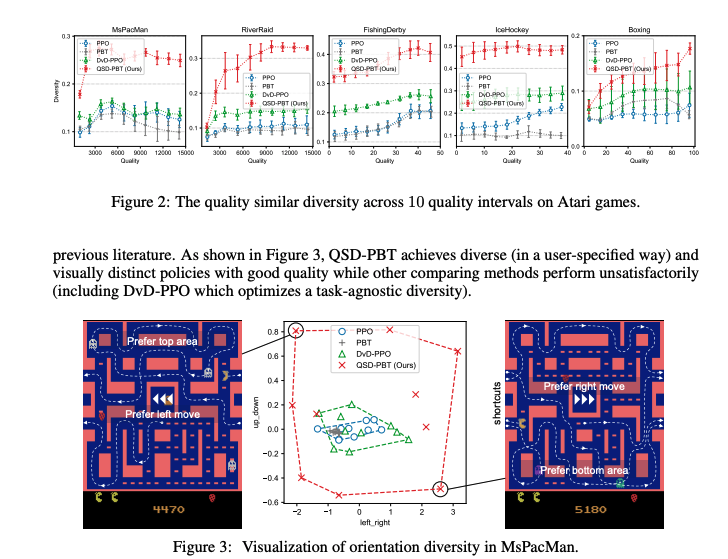
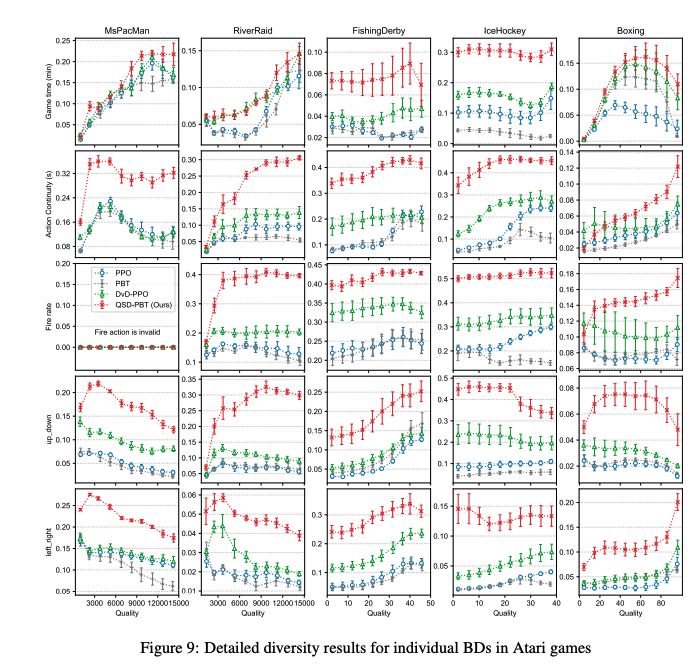