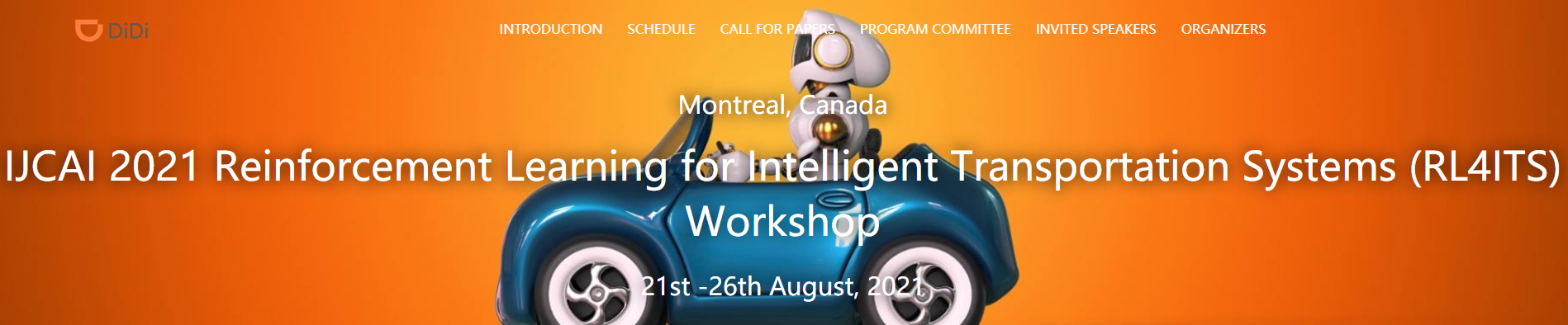
Introduction
Intelligent transportation systems (ITS) are integrated systems that use advanced computer and communication technologies in road transportation, including vehicles, users, and infrastructure, and in traffic and mobility management, as well as for interfaces with other modes of transportation. There are challenging dynamic decision problems and critical questions that readily stand to benefit tremendously from artificial intelligence (AI), for example:
● How should vehicles and passengers be matched in an optimal way to maximize the efficiency of a mobility-on-demand system?
● How do we rebalance/reposition a fleet of vehicles to maximize overall system utilization?
● How can traffic signals adapt intelligently to the dynamic traffic patterns?
● How does an autonomous vehicle respond to human driver behavior in a mixed autonomy traffic?
● What can be done in particular to generate safe control policies in an ITS?
A lot of these problems have a common characteristic that a sequence of decisions are to be made while we care about some cumulative objectives over a length of horizon.
Reinforcement learning (RL) is a machine learning paradigm that trains an agent to learn to take optimal actions (as measured by the total cumulative reward achieved) in an environment through interactions with it and getting feedback signals. It is thus a class of optimization methods for solving sequential decision-making problems. Thanks to the rapid advancement in deep learning research and computing capabilities, the integration of deep neural networks and RL has generated explosive progress in the latter for solving complex large-scale learning problems, attracting huge amount of renewed interests in the recent years. The combination of deep learning and RL has even been considered as a path to general AI. It presents a tremendous potential to solve some hard problems in transportation in an unprecedented way and to have profound positive impact on the daily lives of our community.
RL for ITS is an emerging interdisciplinary area that is undergoing rapid development and still require further understanding on a number of issues, e.g., dynamic environments, run-time performance, and safe deployment.
The main goal of this workshop is to bring together researchers and practitioners from both the RL and transportation communities to answer the following questions:
● What are the recent advances in the state-of-the-art of RL for ITS?
● What are the new application areas in transportation that can be benefited by RL?
● What are the successful stories in real-world applications of RL to ITS?
● What are the challenges and critical issues that may have significant impact on a successful application?
Answering these questions is critical to unleash the potential of the core intelligence in ITS beyond classical machine learning. We hope the participants will leave the workshop with a better idea about the current status of RL for ITS, about what is working well and what is not, thus providing guidance on directions of future research in this exciting area.

Submission
We look for innovative applications of RL and highly related methods, as well as the employment of RL techniques in conjunction with traditional approaches for ITS.
Submission portal: https://cmt3.research.microsoft.com/RL4ITS2021/Submission/Index
