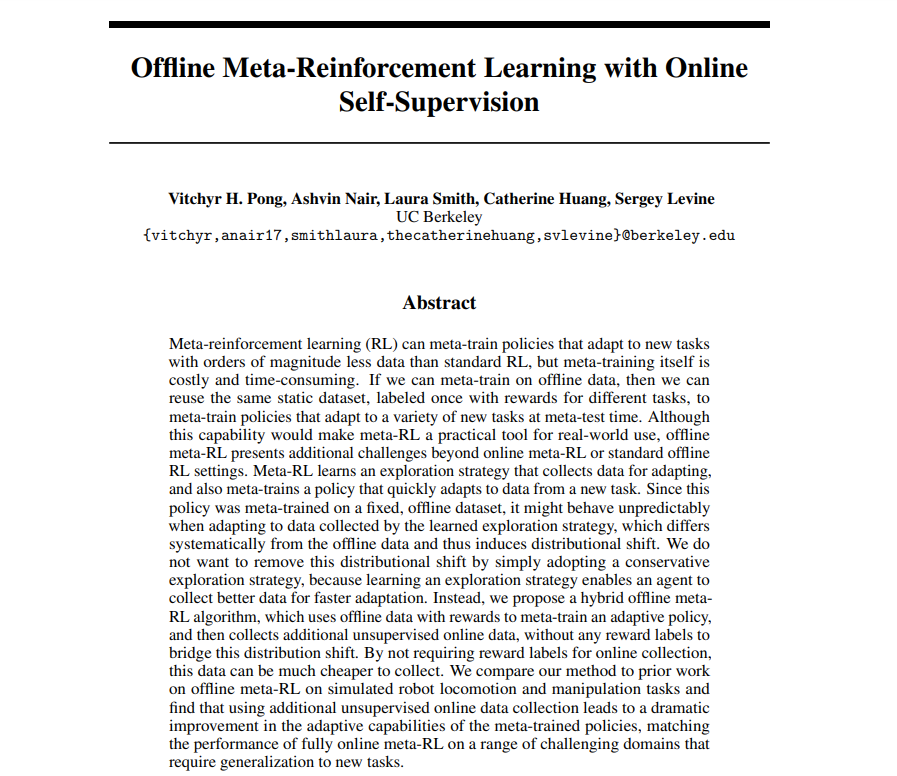
元强化学习 (RL) 可以对适应新任务的策略进行元训练,数据量比标准 RL 少几个数量级,但元训练本身既昂贵又耗时。如果我们可以对离线数据进行元训练,那么我们可以重用相同的静态数据集,标记一次,对不同的任务进行奖励,以在元测试时对适应各种新任务的策略进行元训练。尽管这种能力将使元强化学习成为现实世界使用的实用工具,但离线元强化学习带来了超越在线元强化学习或标准离线强化学习设置的额外挑战。 Meta-RL 学习一种探索策略,该策略收集用于适应的数据,并元训练一种策略以快速适应来自新任务的数据。由于此策略是在固定的离线数据集上进行元训练的,因此在适应由学习探索策略收集的数据时,它可能会出现不可预测的行为,这与离线数据系统地不同,从而导致分布转移。我们不想通过简单地采用保守的探索策略来消除这种分布转变,因为学习探索策略使代理能够收集更好的数据以更快地适应。相反,我们提出了一种混合离线元强化学习算法,它使用带有奖励的离线数据对自适应策略进行元训练,然后收集额外的无监督在线数据,没有任何奖励标签来弥合这种分布转变。由于不需要在线收集奖励标签,因此收集这些数据的成本要低得多。我们将我们的方法与先前在模拟机器人运动和操纵任务上的离线元强化学习的工作进行比较,发现使用额外的无监督在线数据收集导致元训练策略的自适应能力的显着提高,匹配完全在线的性能元强化学习在一系列需要泛化到新任务的具有挑战性的领域上。
Arxiv论文地址: https://arxiv.org/pdf/2107.03974.pdf
The Problem
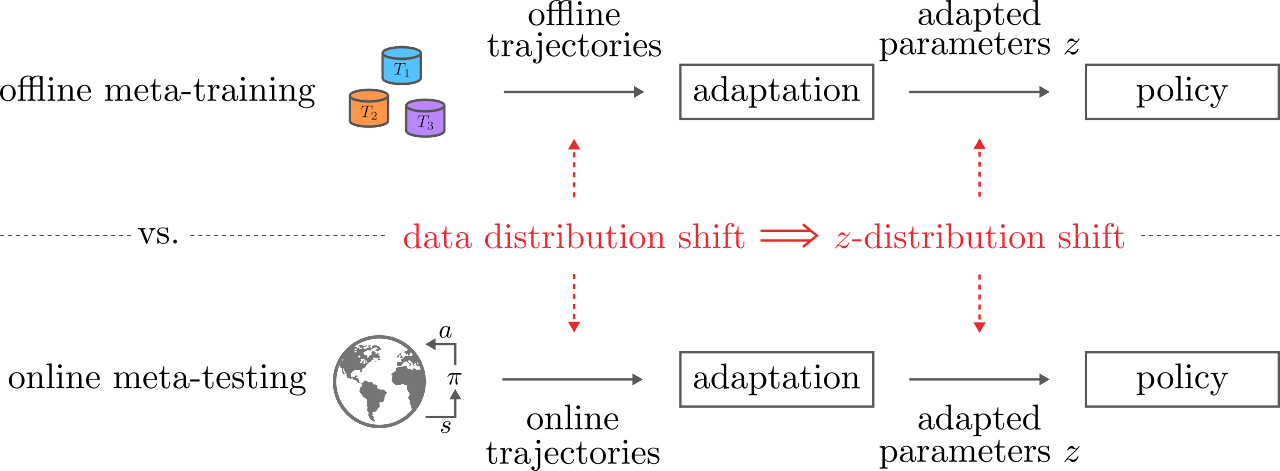
- Meta reinforcement learning (RL) trains a meta-policy to quickly adapt to a new task, given transition histories collected by a learned exploration policy.
- Offline meta RL trains the exploration and meta-policy using a fixed data-set of transitions. However, at meta-test time, the exploration policy's trajectory distribution may differ from the states in the offline replay buffer, resulting in a distribution shift in the data used for adaptation.
- Adaptation data distribution shifts results in distribution shift for the meta-learned context produced by the adaptation procedure.
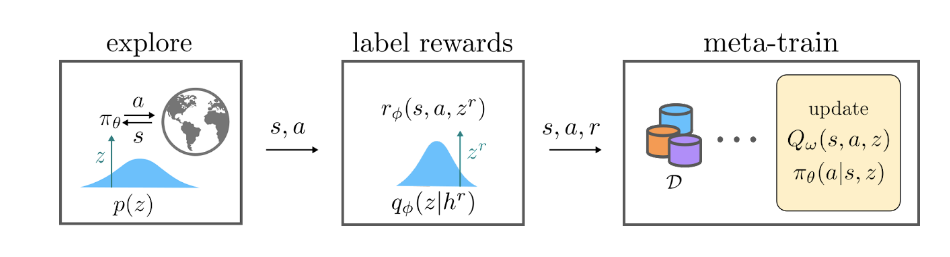
Our Approach: Self-supervised Meta Actor-Critic (SMAC)
- We propose a two stage training procedure that addresses the distribution shift, by performing offline meta RL training followed by a self-supervised phase.
- In the self-supervised phase, the meta-policy can interact with the environment but without additional reward labels.
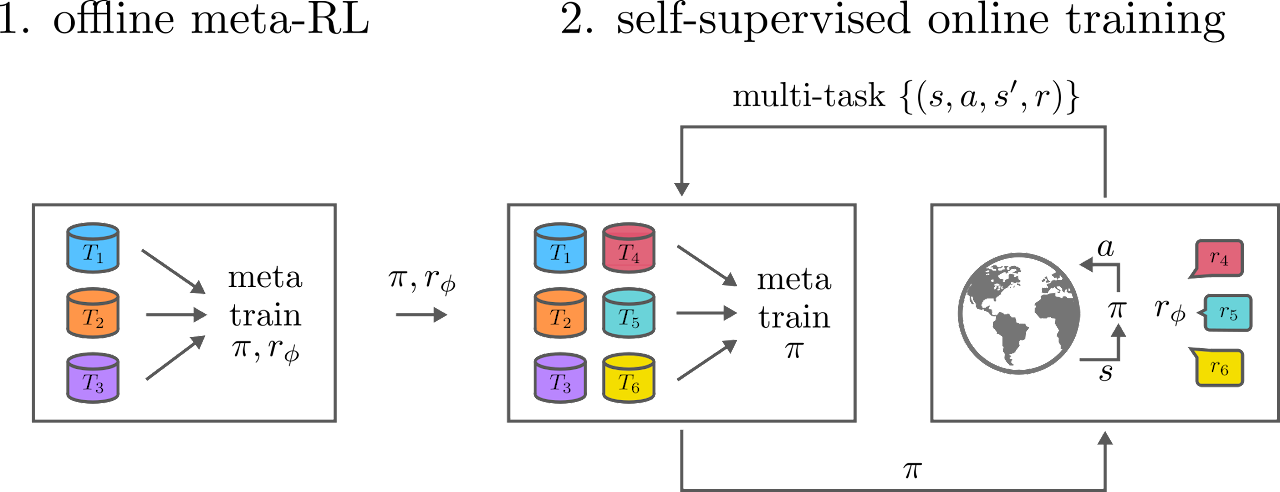
Evaluation
- We propose a new meta-learning evaluation domain based on the environment from this paper.
- A simulated Sawyer gripper can perform various manipulation tasks such as pushing a button, opening drawers, and picking and placing objects.
- We evaluate on held-out tasks in which different objects may be present and with completely different locations.
- See example offline trajectories.
- We also evaluate on standard meta-learning tasks (Cheetah & Ant) .
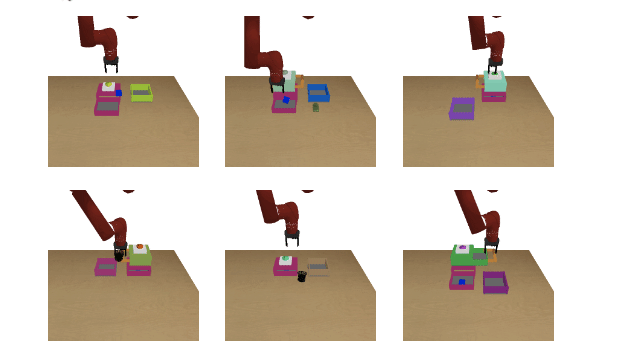
Results
- SMAC significantly improves performance over prior offline meta RL methods.
- SMAC matches performance of oracle comparison that receives reward labels even during the online interactions.
More on the Distribution Shift Problem
Offline RL algorithms suffer from the RL distribution shift where the states seen by the learned policy differ from the states in the offline replay buffer that the policy was trained on, but offline meta RL must deal with an additional distribution shift that arises from the adaptation procedure. Meta RL algorithms learn a fast adaptation procedure that map a history of transitions to a context variable z. Different meta RL algorithms have different representations this context variable: z could be the weights of a neural network, the hidden activations of an RNN, or latent variables generated by a neural network. The commonality of these meta RL methods have is that this context is used to condition a post-adaptation policy. In offline meta-RL, the adaptation procedure is trained using data sampled from a fixed, offline dataset. At meta-test time, the distribution of data that the learned exploration policy collects will differ from the states in the offline replay buffer, and the resulting distribution over z will change. We hypothesize that the policy will perform poorly at meta-test time due to the distribution shift in z-space. To test this hypothesis, we quantify this distribution shift and measure the difference in performance.
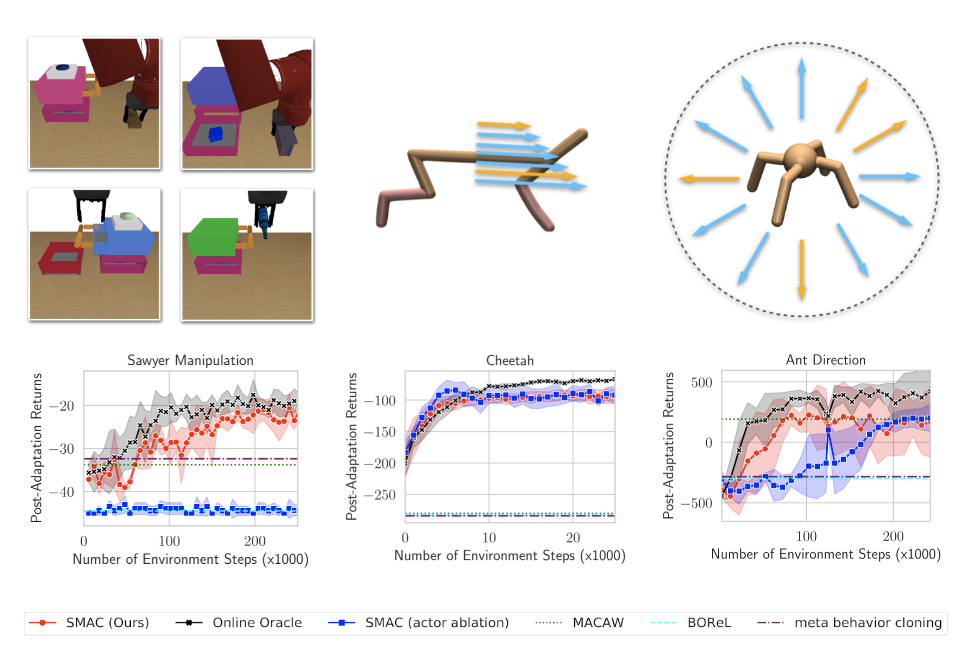
欢迎大家留言讨论