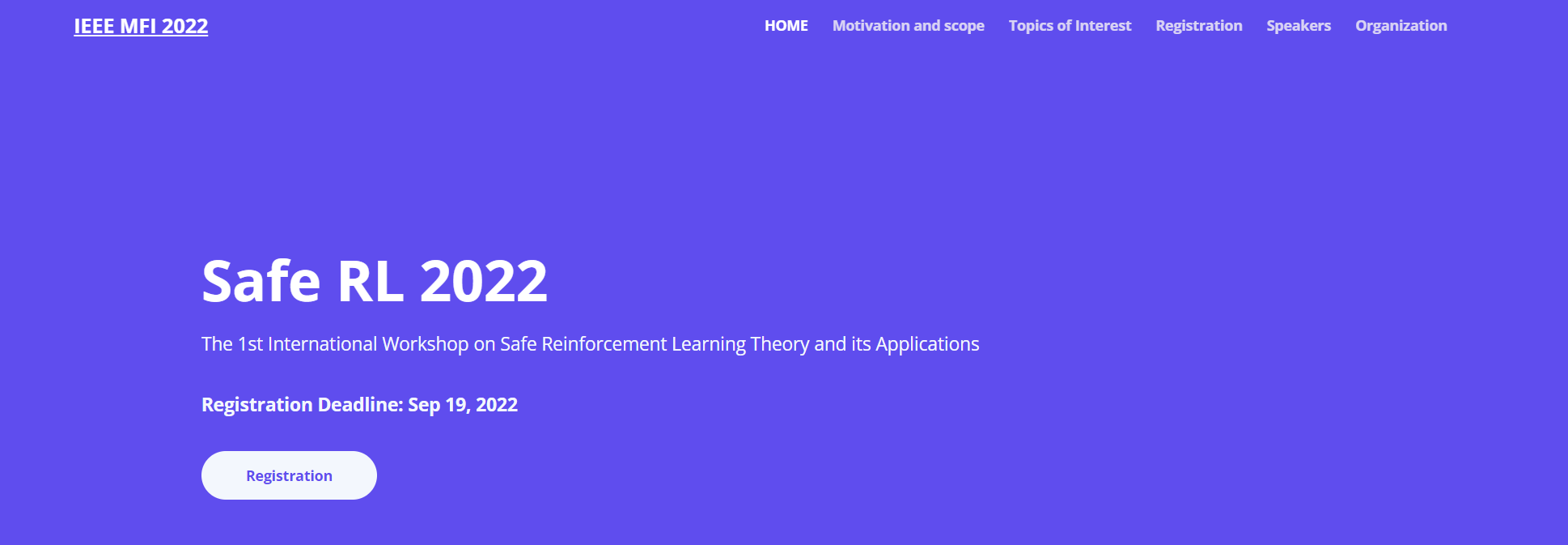
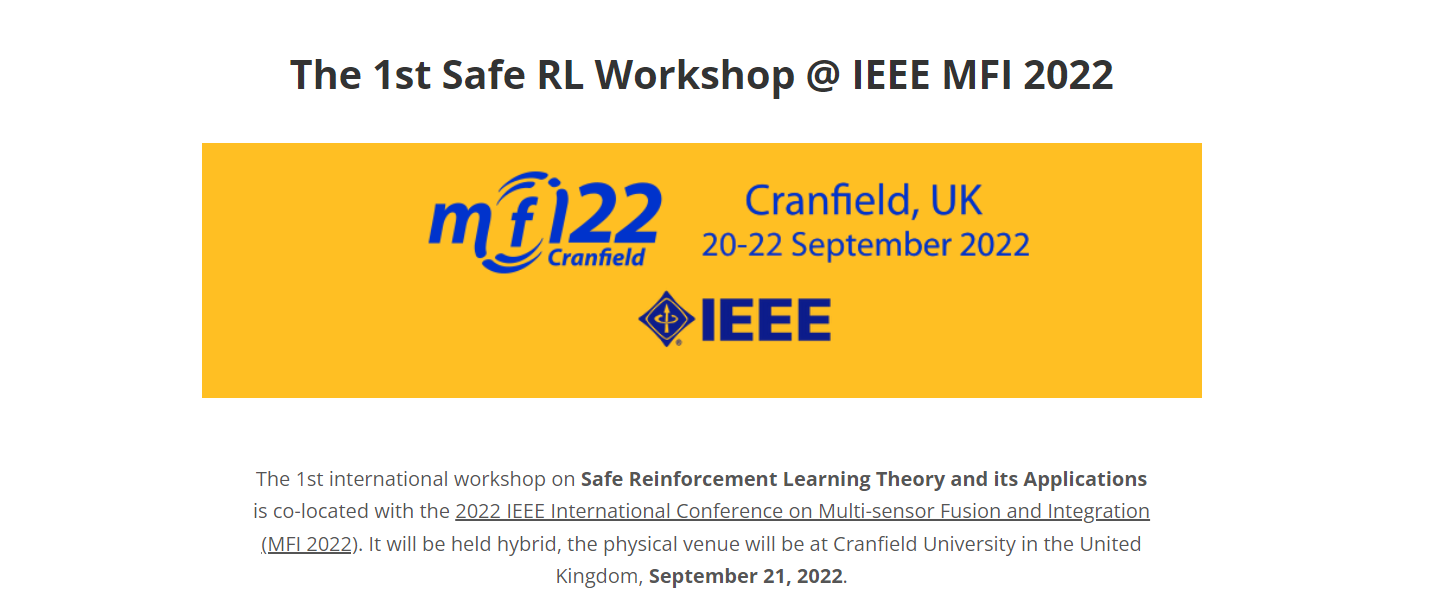
Motivation and Scope
Developing reinforcement learning (RL) algorithms that satisfy safety constraints are becoming increasingly important in real-world applications, which has received substantial attention in recent years. Many methods and algorithms have been proposed and developed for Safe RL, however, how to ensure safety during deploying RL methods in real-world applications and how to develop safe RL in the future still need further discussion. It’s necessary to organize the Safe RL Workshop and discuss how to address safe RL problems with researchers from academia and industry.
Topics of Interest
Areas of interest include, but are not limited to:
Safe reinforcement learning (including single agent and multi-agent RL)
Metrics for safe real-world deployments
Robust reinforcement learning
Decision making under uncertainty
Safe robot learning dataset, simulators, and benchmarks
Robust perception, planning and control
Uncertainty quantification
Safety analysis and safety verification
Transfer learning
Sim2real transfer
Embodied systems and safety-critical applications
Explainability, transparency, and interpretability of learning
Repeatability and reliability of learning-based control
Safe multi-task learning and meta learning